TLDR
Keyword/keyphrase extraction with zero-shot and few-shot semi-supervised domain adaptation.
About AdaptKeyBERT
AdaptKeyBERT expands on KeyBERT by integrating semi-supervised attention for creating a few-shot domain adaptation technique for keyphrase extraction. It also extends the work by allowing zero-shot word seeding, enabling better performance on topic-relevant documents.
Our Aim
- Reconsider downstream training keyword extractors on varied domains by integrating pre-trained LLMs with Few-Shot and Zero-Shot paradigms for domain accommodation.
- Demonstrate two experimental settings with the objectives of achieving high performance for Few-Shot Domain Adaptation & Zero-Shot Domain Adaptation.
- Open source a Python library (AdaptKeyBERT) for the construction of FSL/ZSL for keyword extraction models that employ LLMs directly integrated with the KeyBERT API.
Our Pipeline
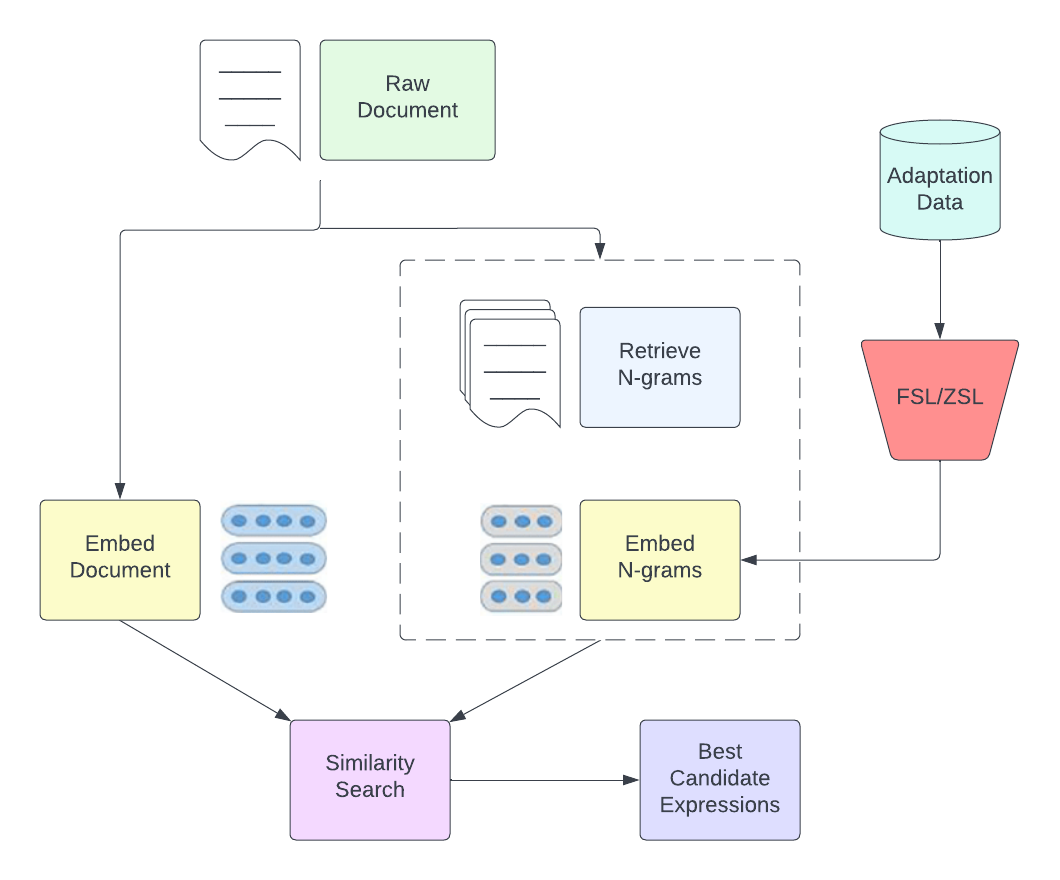
Results
FAO-780 Dataset (p%=10%)
Model | Precision | Recall | F-Score |
---|---|---|---|
Benchmark | 36.74 | 33.67 | 35.138 |
Zero-Shot | 37.25 | 38.59 | 37.908 |
Few-Shot | 40.03 | 39.1 | 39.559 |
Zero-Shot & Few-Shot | 40.02 | 39.86 | 39.938 |
CERN-290 Dataset (p%=10%)
Model | Precision | Recall | F-Score |
---|---|---|---|
Benchmark | 24.74 | 26.58 | 25.627 |
Zero-Shot | 27.35 | 25.9 | 26.605 |
Few-Shot | 29.00 | 27.4 | 28.177 |
Zero-Shot & Few-Shot | 29.11 | 28.67 | 28.883 |
Installation
pip install adaptkeybert
Usage Example
from adaptkeybert import KeyBERT
doc = """
Supervised learning is the machine learning task of learning a function that
maps an input to an output based on example input-output pairs. It infers a
function from labeled training data consisting of a set of training examples.
In supervised learning, each example is a pair consisting of an input object
(typically a vector) and a desired output value (also called the supervisory signal).
A supervised learning algorithm analyzes the training data and produces an inferred function,
which can be used for mapping new examples. An optimal scenario will allow for the
algorithm to correctly determine the class labels for unseen instances. This requires
the learning algorithm to generalize from the training data to unseen situations in a
'reasonable' way (see inductive bias). But then what about supervision and unsupervision, what happens to unsupervised learning.
"""
kw_model = KeyBERT()
keywords = kw_model.extract_keywords(doc, top_n=10) # Usage with candidates - kw_model.extract_keywords(sentence, candidates=candidates, stop_words=None, min_df=1)
print(keywords)
kw_model = KeyBERT(domain_adapt=True)
kw_model.pre_train([doc], [['supervised', 'unsupervised']], lr=1e-3)
keywords = kw_model.extract_keywords(doc, top_n=10)
print(keywords)
kw_model = KeyBERT(zero_adapt=True)
kw_model.zeroshot_pre_train(['supervised', 'unsupervised'], adaptive_thr=0.15)
keywords = kw_model.extract_keywords(doc, top_n=10)
print(keywords)
kw_model = KeyBERT(domain_adapt=True, zero_adapt=True)
kw_model.pre_train([doc], [['supervised', 'unsupervised']], lr=1e-3)
kw_model.zeroshot_pre_train(['supervised', 'unsupervised'], adaptive_thr=0.15)
keywords = kw_model.extract_keywords(doc, top_n=10)
print(keywords)
Citation
@misc{priyanshu2022adaptkeybertattentionbasedapproachfewshot,
title={AdaptKeyBERT: An Attention-Based approach towards Few-Shot & Zero-Shot Domain Adaptation of KeyBERT},
author={Aman Priyanshu and Supriti Vijay},
year={2022},
eprint={2211.07499},
archivePrefix={arXiv},
primaryClass={cs.CL},
url={https://arxiv.org/abs/2211.07499},
}